3 Things Our Team Learned About A/B Testing The Hard Way, So You don’t Have To
Back in 2020, I joined the web presence team at monday.com. Our goal was to be responsible for, well, the website. We quickly set out to find the best ways to improve our home page, following our Growth approach. In order to do this, we wanted to turn to our trusty friend data.
By now, it seems like everyone has heard of A/B tests: you set up two versions of your page, wait to see which performs better, and see the results to roll in! This sounded like a great way to figure out improvements to our web presence, and we got started right away.
Only one problem –– we didn’t realize how much we didn’t know about A/B testing. I’m here to spare you our pain.
First thing’s first: What is an A/B test?
A/B tests are a method of doing experiments where users are being split into two or more groups at random in order to test a new feature or design.
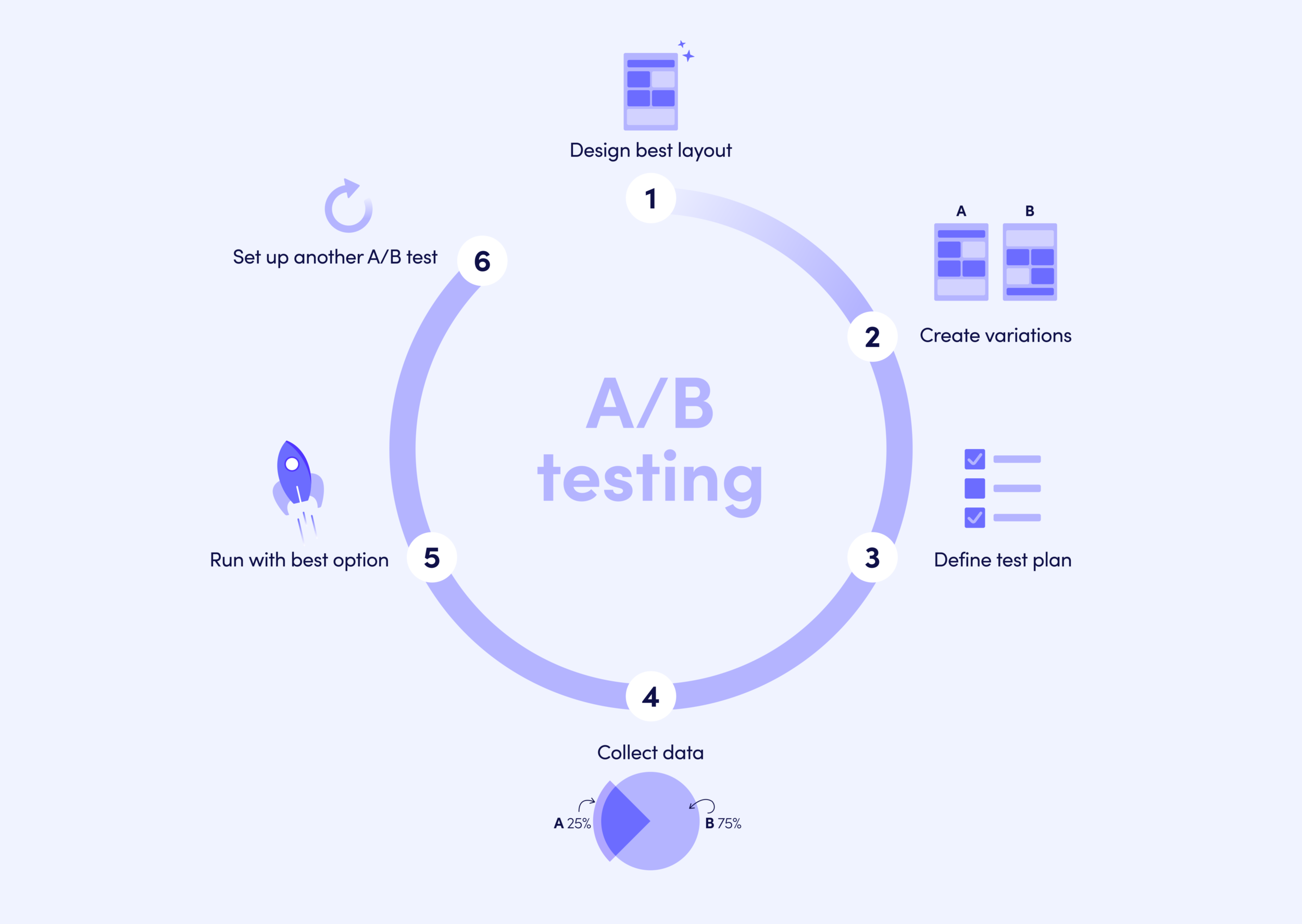
These tests are a statistical analysis that is used to determine which variation performs better for a given metric.
That means you can turn the discussion from ‘we think’ to ‘we know’. They give your team actionable information about your users and how you can improve your product, newsletter, website and more.
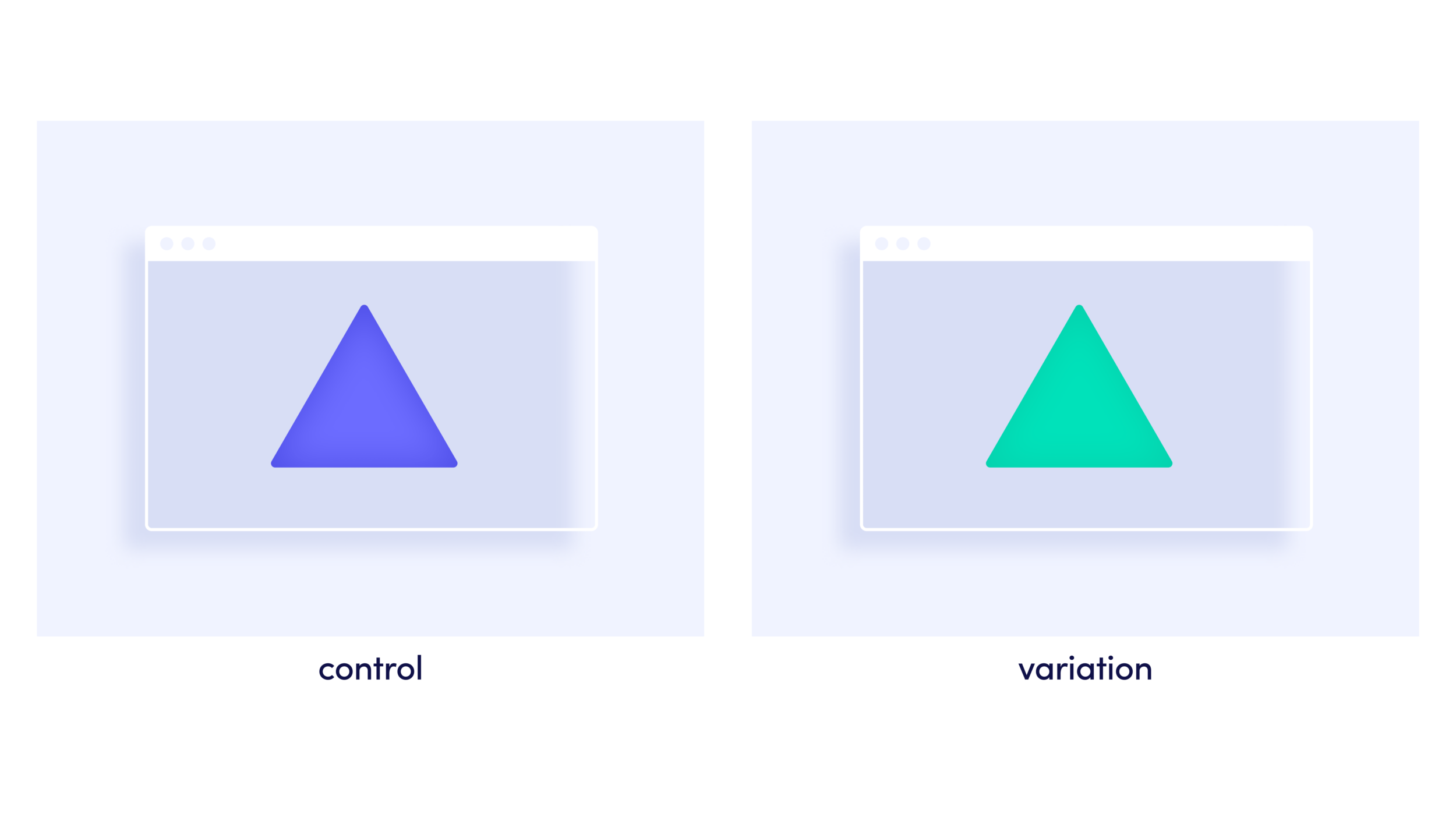
We wanted to show two different versions of our homepage, with visitors to our website being randomly selected to view one or the other in order to see how they would react to what we changed. For example, we wanted to know if changing the shape, from circle to square – will drive more people to click on the tags.
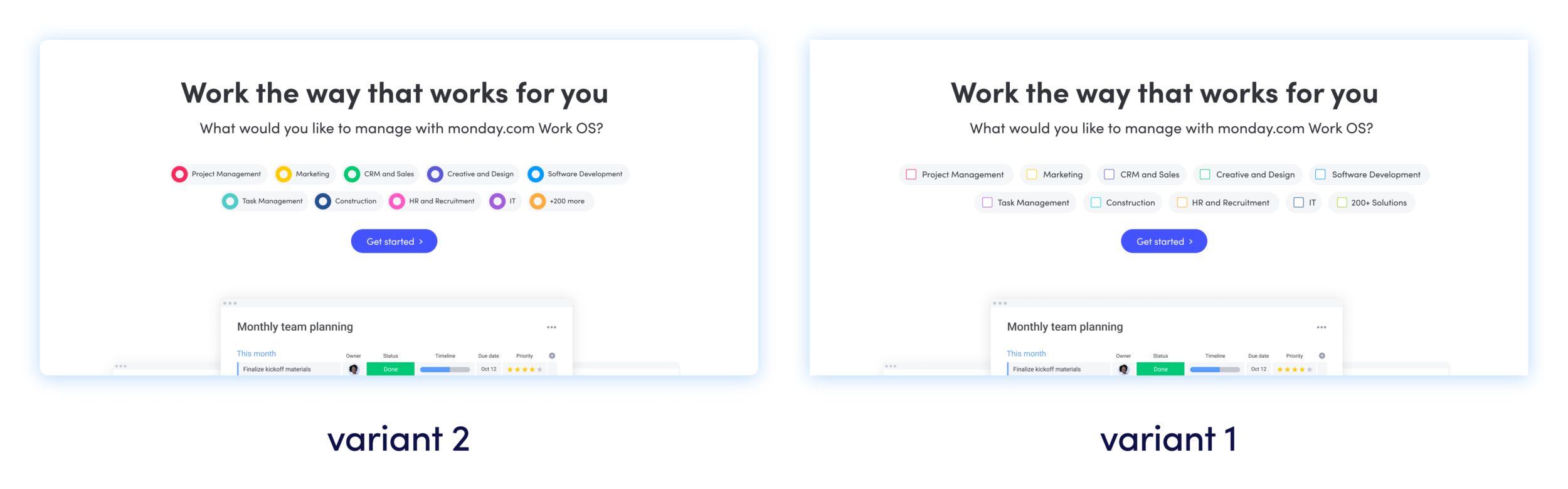
Here’s three things we wish we knew before we started our tests. These insights will help you and your team avoid pitfalls and run tests that actually work.
1. Write an aggressive hypothesis
A hypothesis is a prediction that you make before running a test, and a good hypothesis takes three things:
- Identifying a problem
- Mapping a solution
- Identifying which metric you want to see move.
It should be a bold statement that clearly articulates what change you want to make and why, what you think will change and how.
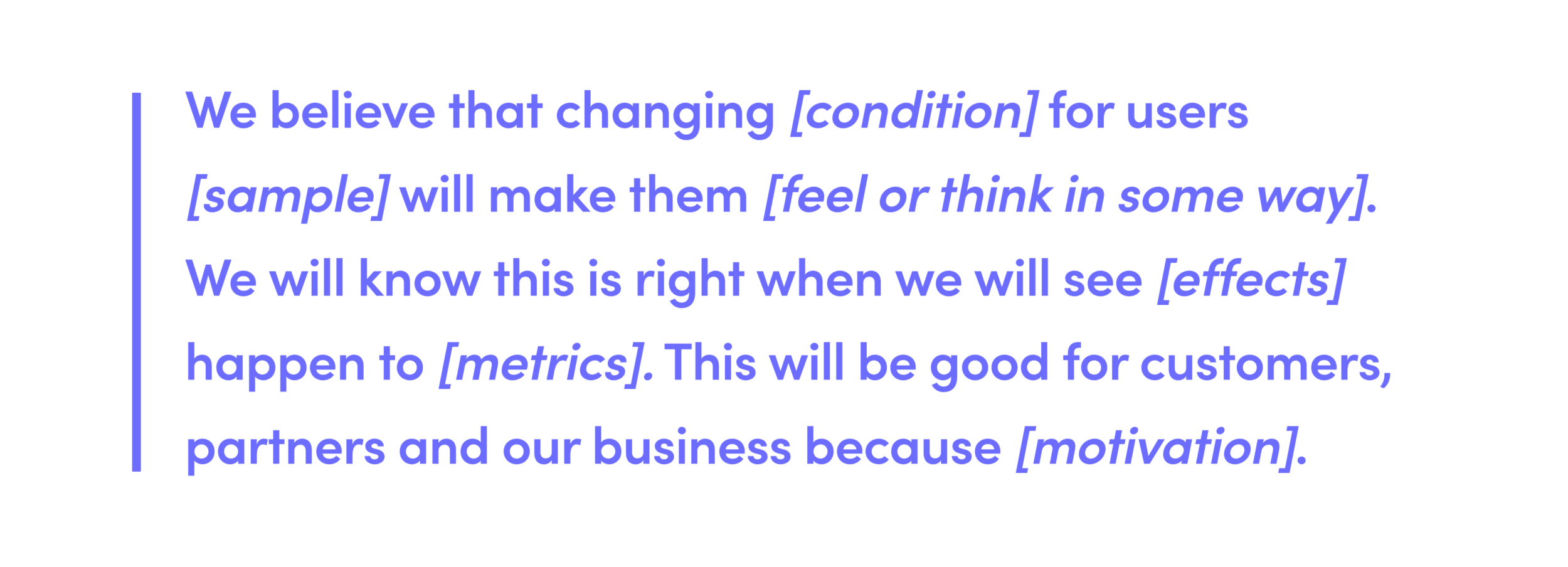
To get the most out of every A/B test, you must think aggressively. A test that loses significantly is much better than a tied test.
Keep in mind that thinking aggressively doesn’t always mean making the biggest change possible –– you can also run a defensive test to see your change isn’t hurting!
2. Stop your test for the right reasons
You should stop an A/B test when your calculations say you have enough data, or it’s clear your execution has failed.
Beware of these two mistakes:
- Stopping early because it seems like one variant overwhelmingly won. Even a “huge win” can become a loss if you let the test gather enough data. Don’t be fooled!
- Stopping late because you are being over-cautious. Letting the test run too long will absorb losses and will skew your results.
Use your calculations and stick to your guns! You can always run a complimentary test to validate results when your valid data is not clear, but stopping at the wrong point is a waste of time.
3. You can still learn from a failed test
It would be amazing if every A/B test produced perfect results. Unfortunately, that isn’t always the case.
At the end of the day, it’s important to remember that there’s no such a thing as losing in an A/B test, as long as you learn from it.
Even if you don’t get the results you want, getting an idea for another A/B test, or even refining how you design and approach your next test is still valuable –– take it from us!
💎 Pro tips
If you made it to this point, good for you! As a reward, here some quick tips to help you make the most out of your A/B testing:
- Start with implementing one change at a time, and save more complicated test variables when you’re comfortable with the basics.
- Consider a KPI that is most likely to be affected by the change, and make a decision about implementing the change based on that.
- Beware of natural variance. Consider running an A/A test to make sure your setup is accurate, and get a better idea of baseline metrics.
- Don’t get emotionally attached to your variant 🙁
- Beware of false positives. Remember: when running 20 tests, 1 can win by chance.
- Running more tests = getting more “chance” wins.
- If you don’t have enough traffic to run an A/B test, use other qualitative and quantitative tools like FullStory, Hotjar, UsabilityHub or UserTesting to gather data instead.
Good luck on your A/B testing and remember to have some fun and be creative. The learning curve will reward you 🙂
If you enjoyed this article, why not check out monday.com’s A/B testing and planning template!